Leveraging Predictive Analytics in Healthcare Decision-Making: A 2024 Guide
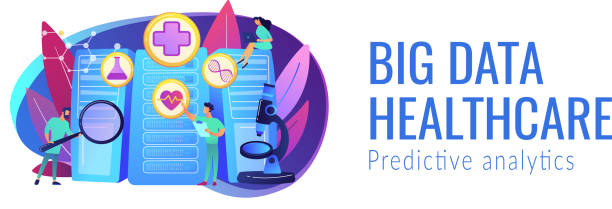
Leveraging Predictive Analytics in Healthcare Decision-Making: A 2024 Guide
Introduction
In 2024, as healthcare continues to embrace technological advancements, predictive analytics emerges as a important tool for enhancing decision-making processes. By analyzing historical data and identifying trends, healthcare leaders can forecast future outcomes, optimize resource allocation, and improve patient care. This blog explores how healthcare executives can effectively leverage predictive analytics to transform their decision-making strategies and achieve superior operational efficiency and clinical outcomes.
The Value of Predictive Analytics in Healthcare
Predictive analytics uses statistical algorithms and machine learning techniques to identify the likelihood of future outcomes based on historical data. In healthcare, this can mean predicting patient influxes, potential health outbreaks, patient health outcomes, and much more. This foresight allows healthcare facilities to prepare more effectively, ensuring they are never caught off guard and can provide the best care possible.
Strategies for Implementing Predictive Analytics:
- Integrate Comprehensive Data Systems:
- Develop integrated data systems that consolidate information from various sources including electronic health records (EHRs), patient management systems, and wearable technology. A unified data repository enhances the quality and accuracy of the data available for analysis.
- Invest in Advanced Analytics Software:
- Utilize sophisticated analytics software capable of handling large datasets and complex algorithms. Ensure the software is scalable and can adapt to the growing and changing data needs of your healthcare facility.
- Train Teams on Data Literacy:
- Cultivate data literacy among healthcare staff to ensure that data-driven insights are correctly interpreted and applied. Training should include understanding basic data analytics concepts, statistical methods, and the implications of predictive analytics findings.
- Employ Data Scientists or Partner with Analytics Firms:
- Hire experienced data scientists who specialize in healthcare analytics or partner with analytics firms. These experts can help develop predictive models that are tailored to the specific needs of your facility.
- Focus on High-Impact Areas:
- Identify areas within your operations where predictive analytics can have the most significant impact, such as patient readmission rates, staffing needs, or inventory management. Starting with high-impact areas can demonstrate quick wins and support broader implementation.
- Implement Real-Time Analytics:
- Deploy real-time analytics to provide ongoing insights that can immediately inform decision-making processes. This is particularly useful in dynamic environments like emergency departments where conditions change rapidly.
- Ensure Regulatory Compliance and Data Privacy:
- Adhere to all applicable regulations concerning data privacy and protection. Implement robust security measures to protect patient data, ensuring that predictive analytics practices comply with laws like HIPAA in the United States.
- Continuously Refine and Validate Models:
- Regularly update and validate predictive models to ensure their accuracy and relevance. Models may degrade over time as patterns change, so continual refinement is necessary.
- Measure Outcomes and ROI:
- Track the outcomes of decisions informed by predictive analytics to assess their effectiveness and calculate return on investment (ROI). This evaluation helps justify the continued use of predictive analytics in healthcare settings.
Challenges in Leveraging Predictive Analytics:
- Complexity of Healthcare Data: The vast and complex nature of healthcare data can make analysis challenging.
- Integration with Existing Systems: Seamlessly integrating new analytics tools with existing IT infrastructure can be technically challenging.
- Cultural Resistance to Data-Driven Decision Making: Shifting from experience-based to data-driven decision-making might meet with resistance from traditional practitioners.
Conclusion
Predictive analytics holds tremendous potential to transform healthcare decision-making in 2024. By effectively implementing these strategies, healthcare leaders can enhance operational efficiencies, improve patient outcomes, and drive innovation within their organizations.
Call to Action
Healthcare executives should consider the strategic implementation of predictive analytics as a cornerstone of their operational strategy. Investing in the right tools, talent, and training will be essential to harnessing the full potential of predictive analytics in healthcare.